使用Python和Numpy进行计算线性代数
本课程于2024年5月发布,由Vetrivel Ravi创建。
MP4 | 视频:h264,1920×1080 | 音频:AAC,44.1千赫 语言:英语 | 大小:1.27 GB | 时长:3小时24分钟
线性代数计算使用NumPy和SciPy,矩阵运算,线性分解,主成分分析
你将学到:
- 学习线性代数的基本原理,如了解其在现实世界中的应用和重要的关键概念
- 了解标量、向量、矩阵和张量之间的区别
- 学习使用Numpy进行矩阵的加法和减法
- 学习使用Numpy进行矩阵乘法
- 学习如何使用Numpy进行矩阵的求逆和转置
- 学习使用Numpy计算矩阵的行列式
- 学习如何使用Numpy计算矩阵的范数、迹和秩
- 学习使用Numpy解决线性方程组
- 学习如何使用Numpy计算特征值和特征向量
- 了解LU、QR和Cholesky分解
- 学习如何使用Numpy创建、切片和重塑张量
- 学习使用线性分解构建电影推荐引擎
- 学习使用奇异值分解构建图像压缩器
- 学习使用线性回归预测房地产市场
- 学习使用非负矩阵分解进行文本挖掘
- 学习使用主成分分析进行降维
要求:
- 无需线性代数的先前经验
- Python和Numpy的基本知识
描述:
欢迎来到《使用Python和Numpy进行计算线性代数》课程。这是一门面向数据科学家和机器学习工程师的综合线性代数教程,该课程将涵盖基本概念、实际实现和实际应用,以增强你在该领域的理解和专业知识。本课程将线性代数与Python完美结合,为那些希望练习编程技能并提高数学知识的人提供了理想的机会。在介绍部分,你将学习线性代数的基本原理,如了解其用例和关键概念。接下来,在下一部分中,我们将开始第一课,你将更多地了解基本概念,如标量、向量和矩阵。此外,你还将学习如何执行矩阵的加法、减法、二乘二矩阵乘法和三乘三矩阵乘法等矩阵运算。随后,在第二课中,你将学习如何手动执行矩阵的求逆和转置,然后你还将学习如何使用Numpy进行计算。在第三课中,你将学习如何手动计算二乘二矩阵和三乘三矩阵的行列式,以及如何使用Numpy进行计算。然后,在第四课中,你将学习如何解决复杂的线性方程组,并确保你理解了概念,我们将尝试许多练习问题。同时,在第五课中,你将学习如何手动计算特征值和特征向量,并使用Numpy进行计算。然后,在第六课中,你将学习关于线性分解,特别是LU、QR和Cholesky分解。首先,我们将手动计算,然后在你理解基本概念后,我们将利用Numpy进行计算。之后,在第七课中,你将学习如何使用Numpy创建具有特定大小的张量,更令人兴奋的是,我们将玩弄张量,并学习如何使用切片和索引技术访问张量的值。然后,在第八课中,你将学习如何手动计算奇异值分解,也使用Numpy进行计算。完成线性代数课程后,我们将确保你有机会将学到的所有概念应用到实际项目中。总共有五个项目,第一个项目中,你将使用线性分解构建推荐引擎,在第二个项目中,你将使用奇异值分解构建图像压缩器,在第三个项目中,你将使用线性回归预测房地产市场,在第四个项目中,你将使用非负矩阵分解进行文本挖掘,在最后一个项目中,你将使用主成分分析进行降维。首先,在开始课程之前,我们需要问自己这个问题,为什么要学习计算线性代数?这是我的答案。线性代数是许多高级数学概念和技术的基础,用于机器学习、数据科学和工程。在机器学习中,线性代数对于理解和实现诸如线性回归、支持向量机和神经网络等算法至关重要。在数据科学中,线性代数使我们能够有效地分析大型数据集、进行降维并解决优化问题。在工程领域,线性代数在建模物理系统、设计控制…
Computational Linear Algebra With Python & Numpy
Published 5/2024
MP4 | Video: h264, 1920×1080 | Audio: AAC, 44.1 KHz
Language: English | Size: 1.27 GB | Duration: 3h 24m
Linear algebra computations using NumPy & SciPy, matrix operations, linear decomposition, principal component analysis
What you’ll learn
Learn the basic fundamentals of linear algebra, such as getting to know its real world applications and important key concepts
Learn about the difference between scalar, vector, matrix, and tensor
Learn how to add and subtract matrix using Numpy
Learn how to multiply matrix using Numpy
Learn how to inverse and transpose matrix using Numpy
Learn how to calculate matrix determinant using Numpy
Learn how to calculate matrix norm, trace, and rank using Numpy
Learn how to solve system of linear equation using Numpy
Learn how to calculate eigenvalues and eigenvectors using Numpy
Learn about LU, QR, and Cholesky decomposition
Learn how to create, slice, and reshape tensor using Numpy
Learn how to build movie recommendation engine using linear decomposition
Learn how to build image compressor using singular value decomposition
Learn how to predict real estate market using linear regression
Learn how to do text mining using non negative matrix factorization
Learn how to perform dimensionality reduction using principal component analysis
Requirements
No previous experience in linear algebra is required
Basic knowledge in Python and Numpy
Description
Welcome to Computational Linear Algebra with Python & Numpy course. This is a comprehensive linear algebra tutorial for data scientists and machine learning engineers, this course will cover fundamental concepts, practical implementations, and real-world applications to enhance your understanding and expertise in the field. This course is a perfect combination between linear algebra and python, making it an ideal opportunity for anyone who is looking to practice their programming skills while improving their mathematical knowledge. In the introduction session, you will learn the basic fundamentals of linear algebra, such as getting to know its use cases and key concepts. Then, in the next section, we will start the first lesson where you will get to know more about the basic concepts like scalar, vector, and matrices. In addition, you will also learn about matrix operations like addition, subtraction, two by two matrix multiplications, and three by three matrix multiplications. Afterward, in the second lesson, you will learn how to perform inverse and transpose on matrices manually, then after that you will also learn how to use Numpy to do the calculation. In the third lesson, you will learn how to calculate determinants of two by two matrices and three by three matrices both manually and using Numpy. Then, in the fourth lesson, you will learn how to solve complex linear equations and to make sure you understand the concepts, we will try many practice problems. Meanwhile, in the fifth lesson, you will learn how to calculate eigenvalues and eigenvectors both manually and using Numpy. Then, in the sixth lesson, you will learn about linear decomposition particularly LU, QR, and Cholesky decomposition. Firstly we will do the calculation manually then after you understand the basic concepts, then we will utilize Numpy for computations. After that, in the seventh lesson, you will learn how to create a tensor with specific size using Numpy and even more exciting, we will play around with the tensors and learn how to access a value of tensor by using slicing and indexing techniques. Then, in the eighth lesson, you will learn how to calculate singular value decomposition both manually and also using Numpy. After we are done with linear algebra lessons, we will make sure that you have the opportunity to implement all concepts that you have learnt into real world projects. In total, there will be five projects, in the first project, you will build recommendation engine using linear decomposition, in the second project, you will build image compressor using singular value decomposition, in the third project, you will predict real estate market using linear regression, in the fourth project, you will do text mining using non negative matrix factorization, and in the last project, you will perform dimensionality reduction using principal component analysis.First of all, before getting into the course, we need to ask ourselves this question, why should we learn about computational linear algebra? Well, here is my answer. Linear algebra serves as the foundation for many advanced mathematical concepts and techniques used in machine learning, data science, and engineering. In machine learning, linear algebra is essential for understanding and implementing algorithms such as linear regression, support vector machines, and neural networks. In data science, linear algebra enables us to analyze large datasets efficiently, perform dimensionality reduction, and solve optimization problems. In engineering, linear algebra plays a critical role in modeling physical systems, designing control systems, and solving differential equations.Below are things that you can expect to learn from this course:Learn the basic fundamentals of linear algebra, such as getting to know its real world applications and important key conceptsLearn about the difference between scalar, vector, matrix, and tensorLearn how to add and subtract matrix using NumpyLearn how to multiply matrix using NumpyLearn how to inverse and transpose matrix using NumpyLearn how to calculate matrix determinant using NumpyLearn how to calculate matrix norm, trace, and rank using NumpyLearn how to solve system of linear equation using NumpyLearn how to calculate eigenvalues and eigenvectors using NumpyLearn about LU, QR, and Cholesky decompositionLearn how to create, slice, and reshape tensor using NumpyLearn how to build movie recommendation engine using linear decompositionLearn how to build image compressor using singular value decompositionLearn how to predict real estate market using linear regressionLearn how to do text mining using non negative matrix factorizationLearn how to perform dimensionality reduction using principal component analysis
Overview
Section 1: Introduction
Lecture 1 Introduction to the Course
Lecture 2 Table of Contents
Lecture 3 Whom This Course is Intended for?
Section 2: Tools & Resources
Lecture 4 Tools & Resources
Section 3: Introduction to Linear Algebra
Lecture 5 Introduction to Linear Algebra
Section 4: Scalar, Vector, Matrix, and Tensor
Lecture 6 Scalar, Vector, Matrix, and Tensor
Section 5: Matrix Addition & Subtraction with Numpy
Lecture 7 Matrix Addition & Subtraction with Numpy
Section 6: Matrix Multiplications with Numpy
Lecture 8 Matrix Multiplications with Numpy
Section 7: Matrix Inverse & Transpose with Numpy
Lecture 9 Matrix Inverse & Transpose with Numpy
Section 8: Calculating Matrix Determinant with Numpy
Lecture 10 Calculating Matrix Determinant with Numpy
Section 9: Calculating Matrix Norm, Trace, and Rank with Numpy
Lecture 11 Calculating Matrix Norm, Trace, and Rank with Numpy
Section 10: Solving System of Linear Equation Using Numpy
Lecture 12 Solving System of Linear Equation Using Numpy
Section 11: Calculating Eigenvalues & Eigenvectors with Numpy
Lecture 13 Calculating Eigenvalues & Eigenvectors with Numpy
Section 12: LU, QR, and Cholesky Decomposition
Lecture 14 LU, QR, and Cholesky Decomposition
Section 13: Creating Tensor with Numpy
Lecture 15 Creating Tensor with Numpy
Section 14: Building Movie Recommendation Engine Using Linear Decomposition
Lecture 16 Building Movie Recommendation Engine Using Linear Decomposition
Section 15: Compressing Image Using Singular Value Decomposition
Lecture 17 Compressing Image Using Singular Value Decomposition
Section 16: Predicting Real Estate Market Using Linear Regression
Lecture 18 Predicting Real Estate Market Using Linear Regression
Section 17: Text Mining Using Non Negative Matrix Factorization
Lecture 19 Text Mining Using Non Negative Matrix Factorization
Section 18: Performing Dimensionality Reduction Using Principle Component Analysis
Lecture 20 Performing Dimensionality Reduction Using Principle Component Analysis
Section 19: Conclusion & Summary
Lecture 21 Conclusion & Summary
People who are interested in learning about linear algebra and its real world applications,People who are interested in data science and machine learning
扫码免费下载
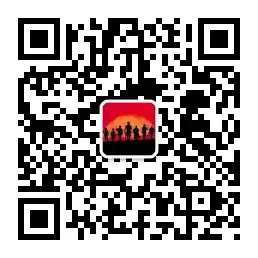