使用Pytorch和CNN检测驾驶汽车速度和空停车位
发布日期:2024年5月 格式:MP4 | 视频:h264, 1920×1080 | 音频:AAC, 44.1 KHz, 2声道 语言:英语 | 时长:2小时54分钟 | 大小:1.26 GB
使用OpenCV、Pytorch、CNN、Keras和SSD构建驾驶汽车速度检测系统和空停车位检测系统。
你将学到:
- 使用OpenCV、Pytorch和单次多框检测器(SSD)构建驾驶汽车速度检测系统
- 使用Keras和卷积神经网络训练空停车位检测系统
- 使用OpenCV构建空停车位检测系统
- 使用OpenCV提取停车位坐标
- 了解驾驶汽车速度检测系统的工作原理,包括车辆检测、轨迹估算、速度计算和速度限制检查
- 了解空停车位检测系统的工作原理,包括数据收集、图像预处理、特征提取和物体检测
- 创建检测速度的函数
- 设置速度限制并检查是否超速
- 创建和发出超速罚单
- 使用OpenCV计算帧率FPS
- 创建统计空停车位数量的函数
- 了解计算机视觉在交通管理中的应用,包括其使用案例、技术限制和将使用的技术
- 使用OpenCV播放视频
- 使用OpenCV检测运动
- 使用OpenCV进行图像处理
- 对驾驶汽车速度和空停车位检测系统进行准确性和性能测试
要求:
- 不需要之前的物体检测经验
- 基本的Python和Pytorch知识
课程描述:
欢迎来到“使用Pytorch和CNN检测驾驶汽车速度和空停车位”课程。这是一门综合性项目课程,逐步指导你如何使用OpenCV、卷积神经网络和Pytorch构建一个先进的驾驶汽车速度检测系统和空停车位检测器。这个课程是计算机视觉和运动检测的完美结合,为你提供了练习编程技能的理想机会,同时将先进的计算机视觉技术整合到交通管理中,并为未来的城市交通创新开辟了道路。
在引言部分,你将了解计算机视觉在交通管理中的应用,包括其使用案例、将使用的技术和一些技术限制。接下来,你将学习驾驶汽车速度检测系统的工作原理,包括车辆检测、轨迹估算、速度计算和速度限制检查。此外,你还将学习空停车位检测系统的工作原理,从数据收集到停车占用分类的全过程。
在开始项目之前,我们将从Kaggle下载一个训练数据集,该数据集包含数百甚至数千张占用和未占用的停车位图像。我们将使用这个数据集训练模型,使其能够区分哪些停车位已被占用,哪些未被占用。
一切准备就绪后,我们将开始项目部分。在第一个部分中,你将逐步学习如何使用OpenCV和Pytorch构建车辆速度检测系统。此外,我们还将设置一个速度限制,所以,每当有车辆超速时,系统会立即向你发送通知并发出超速罚单。同时,在第二个项目中,你将使用OpenCV和卷积神经网络构建一个空停车位检测系统。
一旦我们构建了这些检测系统,我们将进行测试,以确保它们完全正常运行,并且所有编程逻辑都已正确实现。
首先,在进入课程之前,我们需要问自己一个问题:为什么我们要构建驾驶汽车检测系统和空停车位检测系统?关于速度检测系统,其实施可以显著帮助执法机构执行速度限制并提高道路安全。通过准确检测和记录车辆速度,执法人员可以有效识别和处理超速情况,从而降低事故风险,促进安全驾驶行为。此外,速度检测系统收集的数据可以作为起诉交通违规行为的有力证据,确保司机的责任和威慑力。
另一方面,空停车位检测系统为个人和社区提供了诸多好处。通过提供实时的可用停车位信息,该系统有助于减少寻找停车位所浪费的时间,特别是在人口稠密的城市地区。
课程内容:
- 了解计算机视觉在交通管理中的应用,包括其使用案例、技术限制和将使用的技术
- 了解驾驶汽车速度检测系统的工作原理,包括车辆检测、轨迹估算、速度计算、速度限制检查和超速罚单生成
- 了解空停车位检测系统的工作原理,包括数据收集、图像预处理、特征提取、物体检测和占用分类
- 使用OpenCV播放视频
- 使用OpenCV检测运动
- 使用OpenCV进行图像处理
- 创建检测速度的函数
- 使用OpenCV、Pytorch和单次多框检测器构建驾驶汽车速度检测系统
- 设置速度限制并检查是否超速
- 创建和发出超速罚单
- 使用OpenCV计算帧率FPS
- 使用OpenCV构建空停车位检测系统
- 使用Keras和卷积神经网络训练空停车位检测系统
- 创建统计空停车位数量的函数
- 使用OpenCV提取停车位坐标
- 对驾驶汽车速度和空停车位检测系统进行准确性和性能测试
Detecting Car Speed & Empty Parking Spot With Pytorch & Cnn
Published 5/2024
MP4 | Video: h264, 1920×1080 | Audio: AAC, 44.1 KHz
Language: English | Size: 1.26 GB | Duration: 2h 54m
Build car speed detection system and empty parking spot detection system using OpenCV, Pytorch, CNN, Keras, and SSD
What you’ll learn
Learn how to build car speed detection system using OpenCV, Pytorch, and Single Shot Multi Box Detector
Learn how to train empty parking spot detection system using Keras and Convolutional Neural Network
Learn how build empty parking spot detection system using OpenCV
Learn how to extract parking spot coordinate using OpenCV
Learn how a car speed detection system works. This section will cover vehicle detection, trajectory estimation, speed calculation, and speed limit check
Learn how empty parking spot detection systems work. This section will cover data collection, image preprocessing, feature extraction, and object detection
Learn how to create function to detect speed
Learn how to set speed limit and check if the speed exceeds the speed limit
Learn how to create and issue speeding ticket
Learn how to calculate frame rate using OpenCV
Learn how to create function to count how many empty parking spot
Learn about computer vision applications in traffic management, such as getting to know its use cases, technical limitations, and technologies that will be used
Learn how to play video using OpenCV
Learn how to detect motion using OpenCV
Learn how to perform image processing using OpenCV
Learn how to conduct accuracy and performance testing on car speed and empty parking spot detection systems
Requirements
No previous experience in object detection is required
Basic knowledge in Python and Pytorch
Description
Welcome to Detecting Car Speed & Empty Parking Spot with Pytorch & CNN course. This is a comprehensive project based course where you will learn step by step on how to build a cutting edge car speed detection system and empty parking spot finder using OpenCV, Convolutional Neural Network, and Pytorch. This course is a perfect combination between computer vision and motion detection, making it an ideal opportunity for you to practice your programming skills while integrating advanced computer vision technologies into traffic management and also open doors for future innovations in urban transportation. In the introduction session, you will learn about computer vision applications in traffic management, such as getting to know its use cases, technologies that will be used, and some technical limitations. Then, in the next session, you learn how the car speed detection system works? This section will cover vehicle detection, trajectory estimation, speed calculation, and speed limit check. In addition, you will also learn how empty parking lot detection systems work. This section will cover the full process from data collection to parking occupancy classification. Before starting the project, we will download a training dataset from Kaggle, the dataset contains hundreds or even thousands of images of occupied parking lots and unoccupied parking lots. We will use this dataset to train the model to be able to distinguish which parking lot has been occupied and which ones have not been occupied by cars. Once everything is ready, we will start the project section, in the first section, you will be guided step by step on how to build a vehicle speed detection system using OpenCV and Pytorch. In addition to that, we will also set a speed limit, so, whenever there is a car exceeding the speed limit, the system will immediately send you a notification and issue a speeding ticket. Meanwhile, in the second project, you will build an empty parking lot detection system using OpenCV and Convolutional Neural Network. Once we have built those detection systems, we will be conducting testing to make sure that they have been fully functioning and all programming logics have been implemented correctly.First of all, before getting into the course, we need to ask ourselves this question: why should we build a car detection system and empty parking lot detection system? Well, here is my answer, regarding the speed detection system, its implementation can significantly aid law enforcement agencies in enforcing speed limits and enhancing road safety. By accurately detecting and recording vehicle speeds, law enforcement officers can effectively identify and address instances of speeding, thereby reducing the risk of accidents and promoting safer driving behaviors. Moreover, the data collected by the speed detection system can serve as valuable evidence in prosecuting traffic violations, ensuring accountability and deterrence among drivers.On the other hand, the empty parking lot detection system offers numerous benefits to individuals and communities. By providing real-time information on available parking spaces, this system helps to reduce time wasted searching for parking, particularly in densely populated urban areas.Below are things that you can expect to learn from this course:Learn about computer vision applications in traffic management, such as getting to know its use cases, technical limitations, and technologies that will be usedLearn how a car speed detection system works. This section will cover vehicle detection, trajectory estimation, speed calculation, speed limit check, and speed ticket generatorLearn how empty parking spot detection systems work. This section will cover data collection, image preprocessing, feature extraction, object detection, and occupancy classificationLearn how to play video using OpenCVLearn how to detect motion using OpenCVLearn how to perform image processing using OpenCVLearn how to create function to detect speedLearn how to build car speed detection system using OpenCV, Pytorch, and Single Shot Multibox DetectorLearn how to set speed limit and check if the speed exceeds the speed limitLearn how to create and issue speeding ticketLearn how to calculate frame rate using OpenCVLearn how build empty parking spot detection system using OpenCVLearn how to train empty parking spot detection system using Keras and Convolutional Neural NetworkLearn how to create function to count how many empty parking spotLearn how to extract parking spot coordinate using OpenCVLearn how to conduct accuracy and performance testing on car speed and empty parking spot detection systems
Overview
Section 1: Introduction
Lecture 1 Introduction to the Course
Lecture 2 Table of Contents
Lecture 3 Whom This Course is Intended for?
Section 2: Tools, IDE, and Datasets
Lecture 4 Tools, IDE, and Datasets
Section 3: Computer Visions Applications in Traffic Management
Lecture 5 Computer Visions Applications in Traffic Management
Section 4: How Car Speed Detection System Works?
Lecture 6 How Car Speed Detection System Works?
Section 5: How Empty Parking Spot Detection System Works?
Lecture 7 How Empty Parking Spot Detection System Works?
Section 6: Importing OpenCV, Numpy, and Pytorch
Lecture 8 Importing OpenCV, Numpy, and Pytorch
Section 7: Playing Video Using OpenCV
Lecture 9 Playing Video Using OpenCV
Section 8: Detecting Motion Using OpenCV
Lecture 10 Detecting Motion Using OpenCV
Section 9: Creating Function to Calculate Car Speed
Lecture 11 Creating Function to Calculate Car Speed
Section 10: Building Car Speed Detection System with OpenCV, Pytorch, and SSD
Lecture 12 Building Car Speed Detection System with OpenCV, Pytorch, and SSD
Section 11: Setting Speed Limit & Issuing Speeding Ticket
Lecture 13 Setting Speed Limit & Issuing Speeding Ticket
Section 12: Calculating Frame Rate
Lecture 14 Calculating Frame Rate
Section 13: Testing Car Speed Detection System
Lecture 15 Testing Car Speed Detection System
Section 14: Image Processing Using OpenCV
Lecture 16 Image Processing Using OpenCV
Section 15: Finding & Downloading Parking Lot Dataset From Kaggle
Lecture 17 Finding & Downloading Parking Lot Dataset From Kaggle
Section 16: Extracting Parking Spot Coordinates
Lecture 18 Extracting Parking Spot Coordinates
Section 17: Training Empty Parking Spot Detection Model with Keras & CNN
Lecture 19 Training Empty Parking Spot Detection Model with Keras & CNN
Section 18: Building Empty Parking Spot Detection System with OpenCV
Lecture 20 Building Empty Parking Spot Detection System with OpenCV
Section 19: Counting How Many Empty Parking Spot
Lecture 21 Counting How Many Empty Parking Spot
Section 20: Testing Empty Parking Spot Detection System
Lecture 22 Testing Empty Parking Spot Detection System
Section 21: Conclusion & Summary
Lecture 23 Conclusion & Summary
People who are interested in building car speed detection system using OpenCV, Pytorch, and SSD,People who are interested in building empty parking spot detection system using OpenCV, Keras, and CNN
扫码免费下载
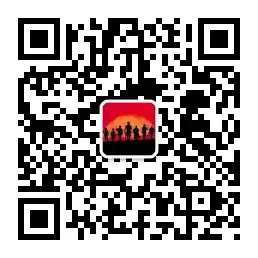